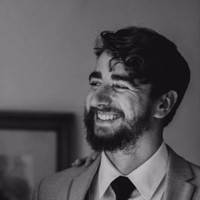
Ionic liquids (ILs) have been identified as a potential alternative to carbonate-based electrolytes that could make next-gen batteries safer and higher in energy/power density. However, their widespread adoption has been precluded by unfavorable transport properties that restrict ion movement at low temperatures and poor long-term stability. The molecular structure space of ILs is vast with an estimated 10^18 possible cation/anion combinations. The inclusion of redox pairs and/or property-tuning additives increases the size of this space even further. Thus, the design and discovery of new IL-based electrolytes could be greatly expedited by high-throughput computational methods that quantitatively predict structure-property relationships (SPRs). I plan to use machine learning, a genetic algorithm and molecular dynamic simulations in tandem to thoroughly explore the solute/IL space and return candidate electrolytes that are electrochemically stable with desirable transport characteristics. Additionally, I hope to gain insight into the intermolecular interactions that govern the SPRs of IL-based electrolytes.
Advisor: Jim Pfaendtner – Chemical Engineering