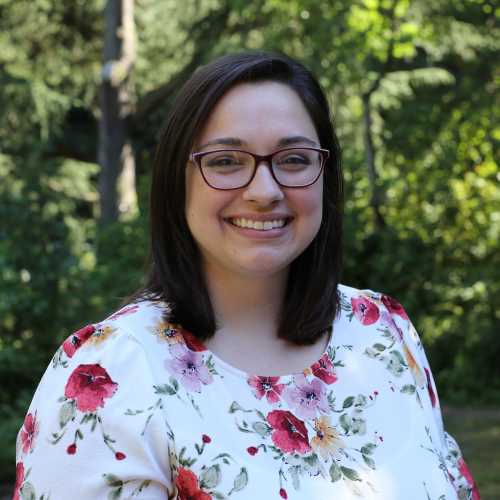
Organic electronics, e.g. photovoltaics, rely on the performance of conjugated polymers (CPs) in their active layers. A better understanding of molecular-macroscopic relationships would enable material design with specific performance criteria, leading to wide-scale implementation of these technologies. Molecular modeling is a powerful tool for probing these systems, but classical modeling parameters, or force fields (FFs), fail to capture electron delocalization effects. Using neutron scattering for validation, our past work demonstrated that even FFs re-parameterized for a model CP, poly(3-hexylthiophene) (P3HT), inconsistently represent backbone behavior at time and length scales relevant to charge-transport mechanisms. Future work will focus on accelerating inefficient FF parameterization and developing improved CP models. Using molecular dynamics simulations of P3HT, we will train a machine learning model to predict neutron scattering profiles from FFs. It will then be used to efficiently explore a parameter search space, within physical bounds, to accurately reproduce experimental neutron scattering data.
Advisor: Lilo Pozzo – Chemical Engineering