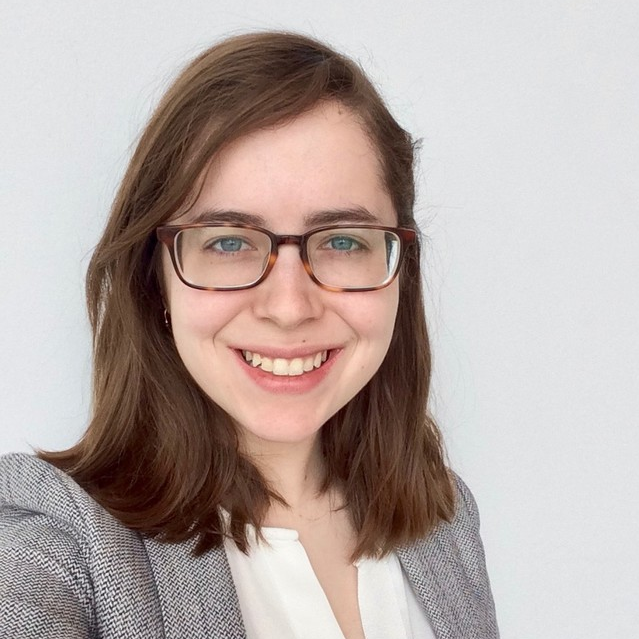
My research focuses on merging data science and machine learning techniques with functional scanning probe microscopy (SPM) to better understand energy materials. We are currently experiencing a convergence of advancement in both data science techniques and scanning probe microscopy resolution and functionality. I aim to take advantage of this confluence to evaluate the spatial heterogeneity of photovoltaic materials. SPM allows us to visualize surface photovoltage, charging rate, chemical composition, and morphology on the nanometer scale. These multimodal techniques produce incredibly dense, multidimensional data that naturally encourage the use of machine learning to extract meaningful information more efficiently. Specifically, I am working on using neural networks to extract the charging constant from time-resolved electrostatic force microscopy data on lead halide perovskites and other photovoltaic materials. With this, and other research, I hope to contribute to the broader understanding of energy material functionality on the nano-scale so we can develop the technologies that will best combat the climate crisis.
Advisor: David Ginger – Chemistry